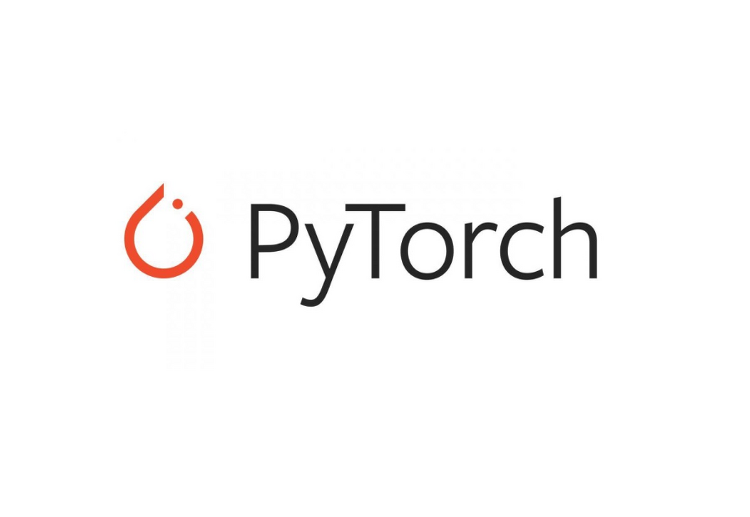
만약 데이터가 GPU를 사용해서 학습을 해야하는 상황이라면 코드 내에서 해당하는 데이터들을 GPU용 텐서로 변환해 주어야 한다. 먼저 device를 정의해주기 !import torchdevice = torch.device("cuda:0" if torch.cuda.is_available() else "cpu") CNN추가추가 RNN데이터 처리시퀀스 데이터를 만드는 함수 내에서 x_seq, y_seq를 변환 후 returny데이터는 view를 사용하여 2차원으로 바꿔준다. 왜냐하면 MSE Loss가 기본적으로 2차원 target data를 받기 때문 !return torch.FloatTensor(x_seq).to(device), torch.FloatTensor(y_seq).to(device)..
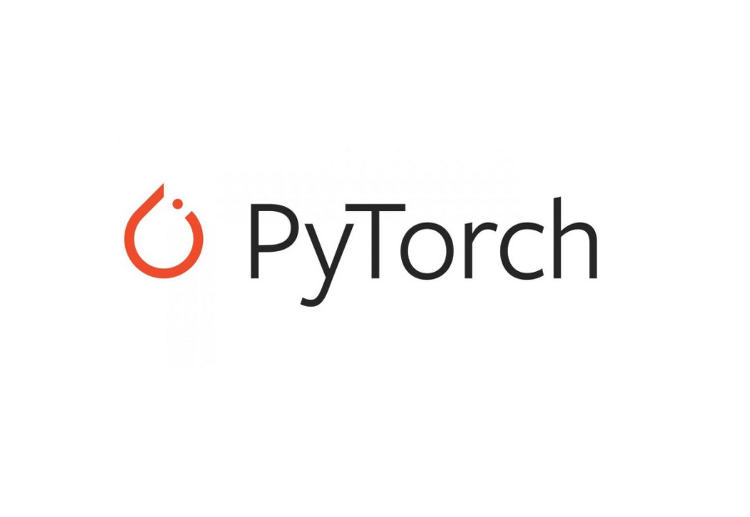
📚 파이토치에는 Dataset과 DataLoader라는 기능이 있어서 미니 배치 학습이나 데이터 셔플, 병렬 처리 까지 간단히 수행할 수 있다.📚 TensorDataset을 DataLoader에 전달해서 데이터의 일부만 간단히 추출할 수 있다. TensorDataset🎀 개념Dataset을 상속한 클래스로 학습 데이터 X와 레이블 Y를 묶어 놓는 컨테이너이다.이것을 DataLoader에 전달하면 for 루프에서 데이터의 일부분만 간단히 추출할 수 있게 된다.TensorDataset에는 텐서만 전달할 수 있으며, Variable은 전달할 수 없으니 주의Dataset은 직접 작성할 수도 있어서 대량의 이미지 파일을 한 번에 메모리에 저장하지 않고 필요할 때마다 읽어서 학습하는 등 다양하게 활용할 수..
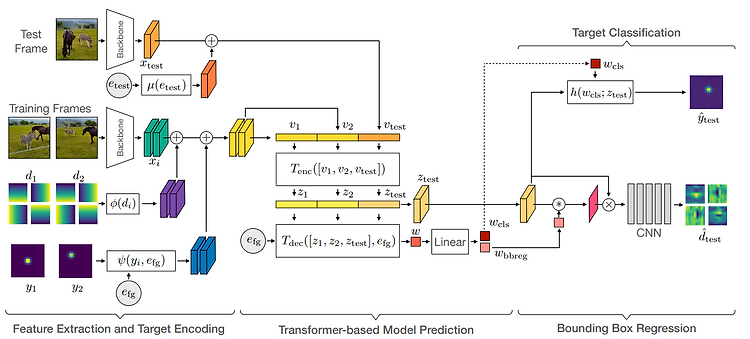
Transforming Model Prediction for Tracking Christoph Mayer, Martin Danelljan, Goutam Bhat, Matthieu Paul, Danda Pani Paudel, Fisher Yu, Luc Van Gool Transforming Model Prediction for Tracking Optimization based tracking methods have been widely successful by integrating a target model prediction module, providing effective global reasoning by minimizing an objective function. While this inductiv..
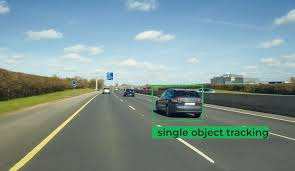
💡 계속 업데이트 예정DCF (Discriminative Correlation Filter)DCF-based 접근법: objective를 최소화함으로써 target과 background를 구별하기 위한 target model을 학습한다. 여러가지 종류들오랫동안 Fourier-transform based solvers는 DCF based trackers [5, 15, 29, 46]에 지배적이었다.[13]은 target model로 two layer Perceptron을 사용하고, 최적화 문제를 해결하기 위해 Conjugate Gradient를 사용한다.최근, 추적 문제를 meta learning problem [1, 58, 72]에 던져 end-to-end training을 가능하게 하는 여러 가지 방..
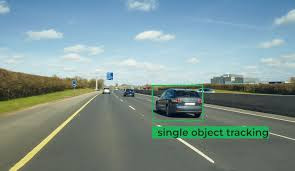
💡 계속 업데이트 예정이 외에도 Transformer를 이용한 트래커들이 많지만, VOT 챌린지에서 EAO 점수가 높은 트래커 위주로 제가 리뷰한 논문을 정리하였습니다. (간단한 모델 아키텍쳐와 특징이나 장점, 단점, 구성을 정리하려고 만든 글입니다. 더 사제한 내용을 보시려면 논문리뷰 포스팅 글을 참고해주세요.) Transformer최근에는 트랜스포머를 사용하는 여러 추적기가 도입되었다.트랜스포머는 일반적으로 target object를 localize & bounding box를 regression 위해 discriminative features를 예측하는 데 사용된다. 인코더: training features 처리디코더: cross-attention layers를 사용하여 training and t..
Bias란 ?Bias타겟과 예측값이 얼마나 멀리 떨어져 있는지 ?Bias⬆ ➡ 데이터로부터 target과의 연관성을 잘 찾아내지 못하는 과소적합 (Underfitting) 문제 발생 Variance예측값들이 얼마나 멀리 퍼져있는지 ?데이터의 사소한 노이즈나 랜덤한 부분까지 민감하게 고려하는 과적합 (Overfitting) 문제 발생Bias를 학습 알고리즘의 잘못된 가정에 의하여 발생하는 오차라고도 하는데잘못된 가정에 의하여 데이터에서 중요한 부분을 놓치고 있다는 의미 💡 우리가 사용하는 ML/DL은 아직 특정 상황에 맞춰 개발된 경우가 대부분이므로, 그 특정 상황에 잘 맞는 일반적인 가정이 있다면 성능을 높일 수 있지 않을까? 하면서 개념이 바로 Inductive Bias Inductive B..
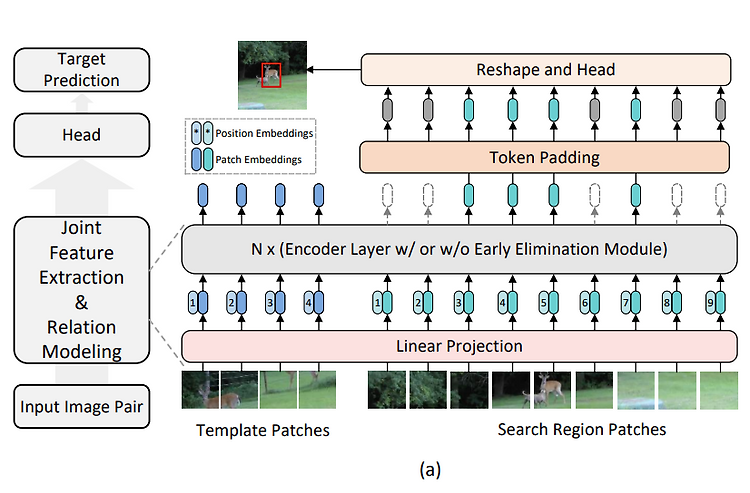
Joint Feature Learning and Relation Modeling for Tracking: A One-Stream FrameworkBotao Ye 1 , 2 , Hong Chang 1 , 2 , Bingpeng Ma 2 , Shiguang Shan 1 , 2 , and Xilin Chen 1 , 2 Joint Feature Learning and Relation Modeling for Tracking: A One-Stream FrameworkThe current popular two-stream, two-stage tracking framework extracts the template and the search region features separately and then perform..
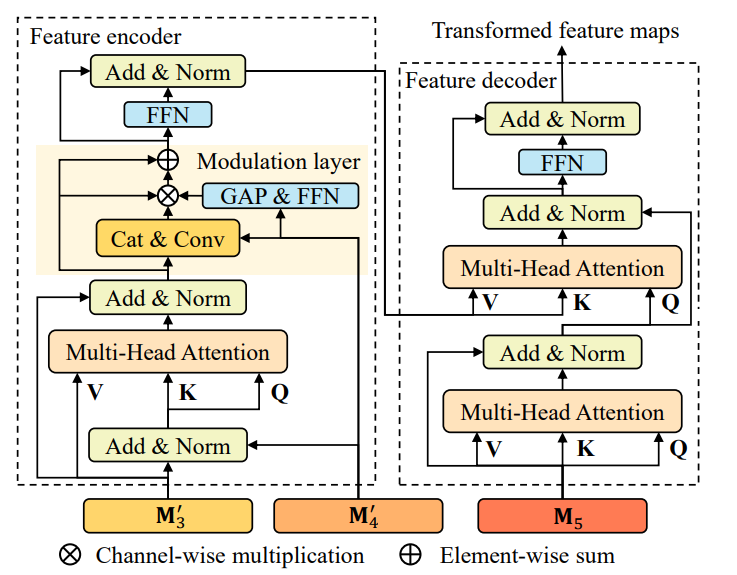
HiFT : Hierarchical Feature Transformer for Aerial Tracking Ziang Cao† , Changhong Fu†,*, Junjie Ye† , Bowen Li† , and Yiming Li‡ HiFT: Hierarchical Feature Transformer for Aerial Tracking Most existing Siamese-based tracking methods execute the classification and regression of the target object based on the similarity maps. However, they either employ a single map from the last convolutional la..
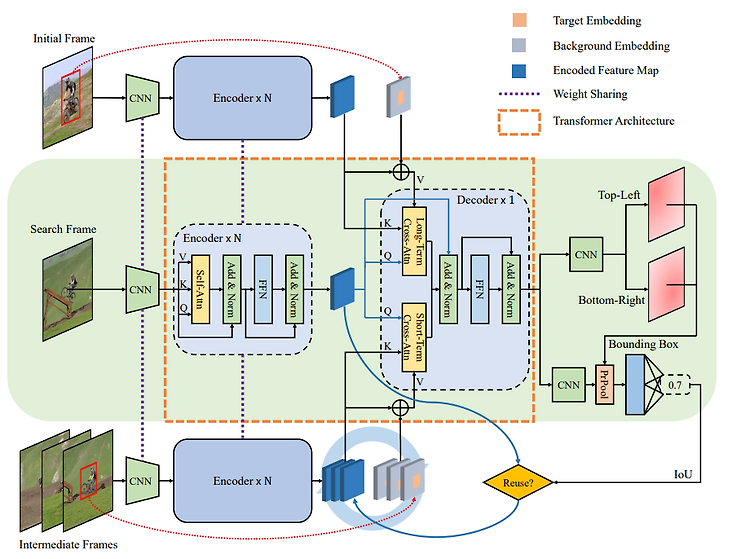
AiATrack : Attention in Attention for Transformer Visual Tracking Shenyuan Gao1 , Chunluan Zhou2 , Chao Ma3 , Xinggang Wang1 , Junsong Yuan4 AiATrack: Attention in Attention for Transformer Visual Tracking Transformer trackers have achieved impressive advancements recently, where the attention mechanism plays an important role. However, the independent correlation computation in the attention me..
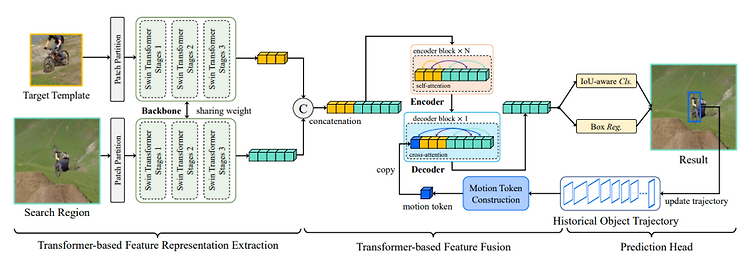
SwinTrack: A Simple and Strong Baseline for Transformer Tracking Liting Lin1,2∗ Heng Fan3∗ Zhipeng Zhang4 Yong Xu1,2 Haibin Ling5 SwinTrack: A Simple and Strong Baseline for Transformer Tracking Recently Transformer has been largely explored in tracking and shown state-of-the-art (SOTA) performance. However, existing efforts mainly focus on fusing and enhancing features generated by convolutiona..